“Why so Many Windows?”
How the ImageNet Image Database Influences Automated Image Recognition of Historical Images
Identifiers (Article)
Abstract
In the field of automated image recognition, computer vision or artificial ‘intelligence,’ the ImageNet data collection plays a central role as a training dataset. For the research project Training The Archive, which aims to make digital humanities methods available for the curating of art, the extent to which ImageNet influences the software prototype The Curator’s Machine is discussed. The Curator’s Machine is designed to facilitate the discovery of relationships and connections between artworks for curators. The text explains how ImageNet, anchored in contemporary image worlds, acts on contemporary and historical artworks by 1) examining the absence of the classification ‘art’ in ImageNet, 2) questioning ImageNet’s lack of historicity, and 3) discussing the relationship between texture and outline in ImageNet-based automated image recognition. This research is important for the genealogical, art historical, and coding related usage of ImageNet in the fields of curating, art history, art studies and digital humanities.
Statistics

References
Alashhab, Samer, Antonio-Javier Gallego, and Miguel Ángel Lozano. 2019. “Hand Gesture Detection with Convolutional Neural Networks.” In Distributed Computing and Artificial Intelligence, 15th International Conference, edited by Fernando De La Prieta, Sigeru Omatu, and Antonio Fernández-Caballero, 45–52. Cham: Springer International Publishing.
Amat, Josep, and Alicia Casals. 1992. “Image Obtention and Preprocessing.” In Computer Vision: Theory and Industrial Applications, edited by Carme Torras, 1–58. Berlin, Heidelberg: Springer Berlin Heidelberg.
Bönisch, Dominik. 2021. “Curator’s Machine – Clustering Museum Collection Data by Annotating Hidden Patterns of Relationships between Artworks.” International Journal for Digital Art History, no. 5 (May): 20–35. doi:10.11588/DAH.2020.5.75953.
Bowyer, Kevin W., Michael C. King, Walter J. Scheirer, and Kushal Vangara. 2020. “The ‘Criminality From Face’ Illusion.” IEEE Transactions on Technology and Society 1 (4): 175–83. doi:10.1109/TTS.2020.3032321.
Broeckmann, Andreas. 2016. Machine Art in the Twentieth Century. Leonardo Book Series. Cambridge, MA: MIT Press.
Brownlee, Jason. 2019. “Best Practices for Preparing and Augmenting Image Data for CNNs.” Blog. Machine Learning Mastery. May 2. https://machinelearningmastery.com/best-practices-for-preparing-and-augmenting-image-data-for-convolutional-neural-networks/.
Burrell, Jenna. 2016. “How the Machine ‘Thinks’ – Understanding Opacity in Machine Learning Algorithms.” Big Data & Society 3 (1). doi:10.1177/2053951715622512.
Cardon, Dominique, Jean-Philippe Cointet, and Antoine Mazieres. 2018. “Neurons Spike Back: The Invention of Inductive Machines and the Artificial Intelligence Controversy.” Reseaux 36 (211): 173–220. doi:10.3917/res.211.0173.
Chaki, Jyotismita, and Nilanjan Dey. 2020. A Beginner’s Guide to Image Preprocessing Techniques. Boca Raton London: CRC PRESS.
Crawford, Kate, and Trevor Paglen. 2019. “Excavating AI – The Politics of Images in Machine Learning Training Sets.” Website. Excavating AI. September 19. https://www.excavating.ai.
Gatys, Leon A., Alexander S. Ecker, and Matthias Bethge. 2015. “A Neural Algorithm of Artistic Style.” ArXiv:1508.06576 [Cs, q-Bio], September. http://arxiv.org/abs/1508.06576.
Geirhos, Robert, Patricia Rubisch, Claudio Michaelis, Matthias Bethge, Felix A. Wichmann, and Wieland Brendel. 2019. “ImageNet-Trained CNNs Are Biased towards Texture – Increasing Shape Bias Improves Accuracy and Robustness.” ArXiv:1811.12231, January. http://arxiv.org/abs/1811.12231.
Goh, Gabriel, Nick Cammarata, Chelsea Voss, Shan Carter, Michael Petrov, Ludwig Schubert, Alec Radford, and Chris Olah. 2021. “Multimodal Neurons in Artificial Neural Networks.” Distill 6 (3). doi:10.23915/distill.00030.
Goodfellow, Ian J., Jonathon Shlens, and Christian Szegedy. 2015. “Explaining and Harnessing Adversarial Examples.” ArXiv:1412.6572, March. http://arxiv.org/abs/1412.6572.
Groys, Boris. 2004. Über Das Neue – Versuch Einer Kulturökonomie. 3rd ed. Fischer Forum Wissenschaft Kultur & Medien. Frankfurt am Main: Fischer-Taschenbuch-Verl.
Hanna, Alex, Emily Denton, Razvan Amironesei, Andrew Smart, and Hilary Nicole. 2020. “Lines of Sight.” Online Magazin. Logic Magazine. December. https://logicmag.io/commons/lines-of-sight/.
Huang, Xun, and Serge Belongie. 2017. “Arbitrary Style Transfer in Real-Time with Adaptive Instance Normalization.” ArXiv:1703.06868, July. http://arxiv.org/abs/1703.06868.
Huh, Minyoung, Pulkit Agrawal, and Alexei A. Efros. 2016. “What Makes ImageNet Good for Transfer Learning?” ArXiv:1608.08614 [Cs], December. http://arxiv.org/abs/1608.08614.
Kornblith, Simon, Jonathon Shlens, and Quoc V. Le. 2019. “Do Better ImageNet Models Transfer Better?” ArXiv:1805.08974 [Cs, Stat], June. http://arxiv.org/abs/1805.08974.
Krizhevsky, Alex, Ilya Sutskever, and Geoffrey E. Hinton. 2012. “ImageNet Classification with Deep Convolutional Neural Networks.” In Proceedings of the 25th International Conference on Neural Information Processing Systems - Volume 1, 1097–1105. NIPS’12. USA: Curran Associates Inc.
Kunsthistorisches Institut der CAU Kiel. 2019. “Fachausdrückezur Benennung Und Beschreibung von Gewandfalten Figürlicher Darstellungen–Bildkünste.” CAU Kiel. https://www.kunstgeschichte.uni-kiel.de/de/infos-fuer-das-studium/fachausdrucke-bildkunste-ss-2019-neu.pdf.
Lee, Rosemary. 2020. “Machine Learning and Notions of the Image.” Dissertation, Copenhagen: Center for Computer Games Research, Department of Digital Design, IT-University of Copenhagen. https://en.itu.dk/~/media/en/research/phd-programme/phd-defences/2020/phd-thesis-final-version-rosemary-lee-pdf.pdf?la=en.
Li, Fei-Fei, Jia Deng, Wei Dong, Richard Socher, Li-Jia Li, and Kai Li. 2009. “ImageNet: A Large-Scale Hierarchical Image Database.” In 2009 IEEE Conference on Computer Vision and Pattern Recognition, 248–55. Miami, FL: IEEE. doi:10.1109/CVPR.2009.5206848.
Malevé, Nicolas. 2020. “On the Data Set’s Ruins.” AI & SOCIETY. doi:10.1007/s00146-020-01093-w.
Munn, Luke. 2020. Logic of Feeling – Technology’s Quest to Capitalize Emotion. Lanham: Rowman & Littlefield.
Offert, Fabian, and Peter Bell. 2020. “Perceptual Bias and Technical Metapictures – Critical Machine Vision as a Humanities Challenge.” AI & SOCIETY, October. doi:10.1007/s00146-020-01058-z.
Parikka, Jussi. 2021. “On Seeing Where There’s Nothing to See – Practices of Light beyond Photography.” In Photography off the Scale – Technologies and Theories of the Mass Image, edited by Jussi Parikka, 185–210. Edinburgh: Edinburgh University Press.
Pasquinelli, Matteo. 2019. “How a Machine Learns and Fails – A Grammar of Error for Artificial Intelligence.” Spheres. Journal for Digital Cultures., no. 5 (November): 1–17.
Pereira, Gabriel, and Bruno Moreschi. 2020. “Artificial Intelligence and Institutional Critique 20 – Unexpected Ways of Seeing with Computer Vision.” AI & SOCIETY, September. doi:10.1007/s00146-020-01059-y.
Rosenblatt, Frank. 1957. “The Perceptron – A Perceiving and Recognizing Automation.” 85-460–1. Buffalo, NY: Cornell Aeronautical Laboratory.
Sauerländer, Willibald. 1970. Gothic Sculpture in France, 1140-1270. New York, NY: Harry N. Abrams.
Schmitt, Philipp. 2019. “Declassifier.” Website. Humans of AI. 2020. https://humans-of.ai/.
Stack, John. 2019. “What the Machine Saw.” Website. What the Machine Saw. https://johnstack.github.io/what-the-machine-saw/index.html.
Stinson, Catherine. 2020. “The Dark Past of Algorithms That Associate Appearance and Criminality – Machine Learning That Links Personality and Physical Traits Warrants Critical Review.” Online Publication. American Scientist. December 2. https://www.americanscientist.org/article/the-dark-past-of-algorithms-that-associate-appearance-and-criminality.
Yosinski, Jason, Jeff Clune, Yoshua Bengio, and Hod Lipson. 2014. “How Transferable Are Features in Deep Neural Networks?” ArXiv:1411.1792 [Cs], November. http://arxiv.org/abs/1411.1792.
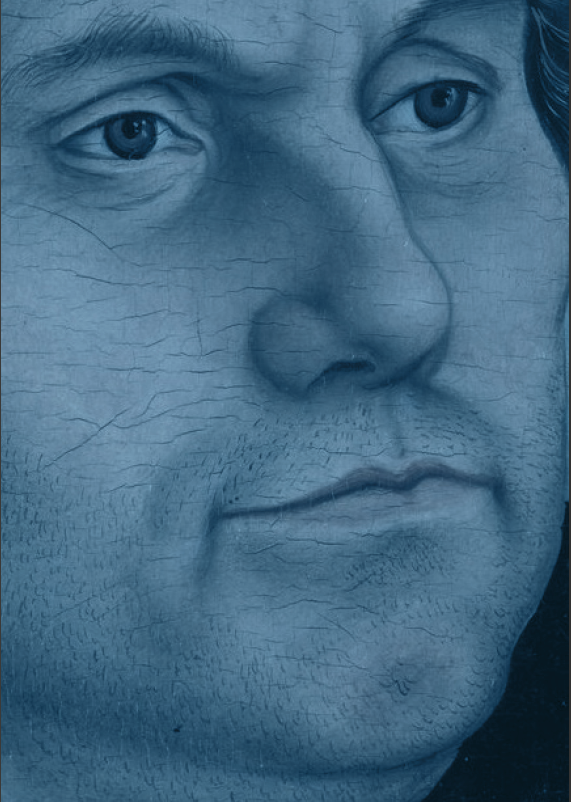